One of the most valuable attributes of GreyNoise is the ability to increase Security Operations Center efficiency by providing context, which allows the relevant security personnel to prioritize alerts. We listen and collect data from around the world from IP addresses that are opportunistically scanning the internet. We intentionally do not solicit traffic and rotate the IPs our services listen on frequently to preserve one of the most important attributes of the GreyNoise dataset: All traffic we observe is unsolicited in nature.
This attribute of our dataset allows us to quickly provide contextual information to answer the question, “Is my organization the victim of a targeted attack?”
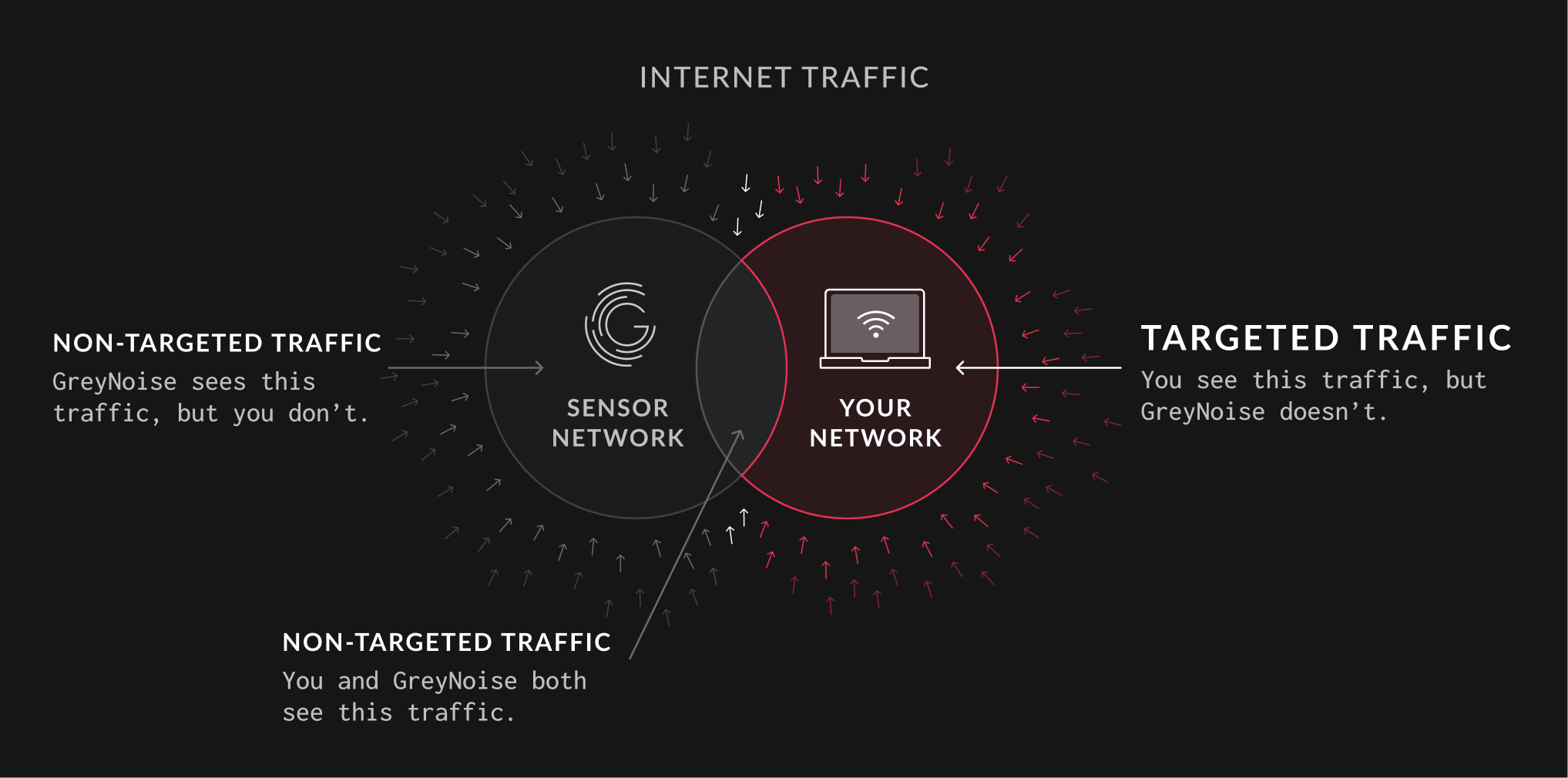
On the left is the IP traffic GreyNoise can observe. On the right is the IP traffic a given organization may observe. Where these two groups overlap is the traffic seen by both GreyNoise and your organization. Unfortunately, this means that targeted network requests to your organization may frequently be outside of what GreyNoise can see.
If your organization observes traffic from an IP that has not been observed by GreyNoise, this traffic is likely targeted at your organization due to business verticals, software vendors in use, or implied value. Alerts falling into this category deserve a higher priority for investigation.
The largest volume of traffic targeted at your organization will likely be sourced from your desired user base or from automated tooling specific to your organization’s needs, such as API calls. As this is a near-constant occurrence, your security and infrastructure team are best equipped to recognize and identify what qualifies as normal.
Of course, there is also malicious network traffic targeted at your organization that GreyNoise may not have observed. Despite it being targeted, GreyNoise can still help provide context.
Consider that your organization has observed HTTPS traffic to the path “/v2.57/version” from an IP that GreyNoise has not observed. The GreyNoise Query Language (GNQL) supports wildcards, meaning that values and attributes of network requests that are specific to your organization, such as version differences in software, can be omitted in order to return query results that preserve the overall structure of the request.
Example: raw_data.web.paths:"/v*/version"
This allows you to surface IPs and context that GreyNoise has observed that share structural similarities with traffic observed by your organization that GreyNoise has not observed.

While this type of context association is fuzzy in nature, we can still quickly ascertain that the traffic observed by your organization is likely to be targeting web-accessible containerization software.
Since the traffic observed by your organization was HTTPS, you can further combine and pivot stricter fingerprints such as JA3 hashes to rapidly create actionable documentation for investigations of targeted network traffic.
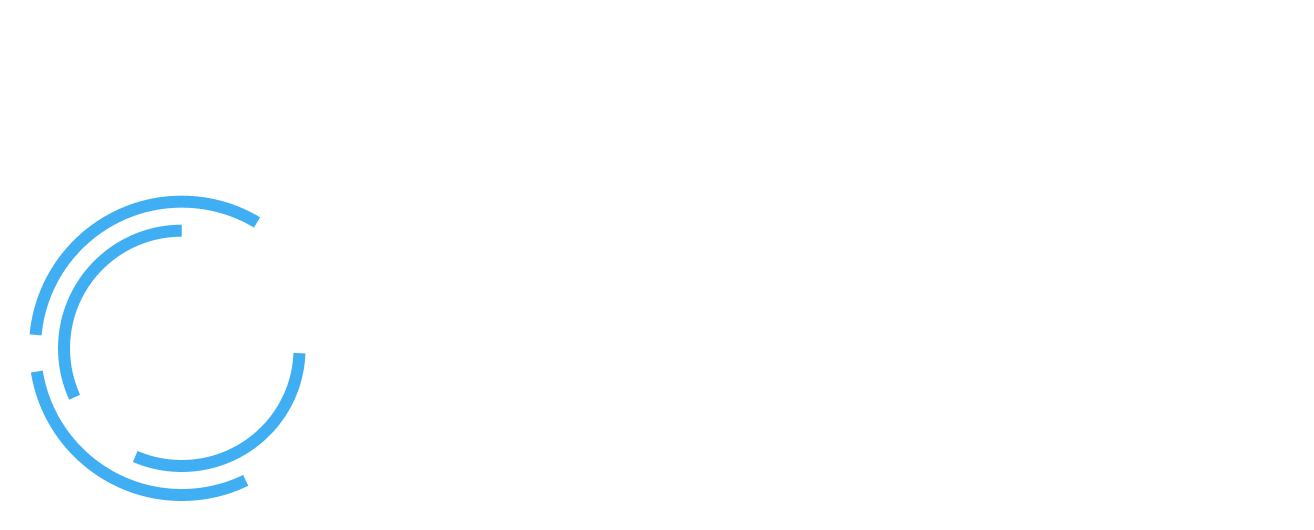